| Home | Research | Coursework | Publications | Others | CV & Contact | |
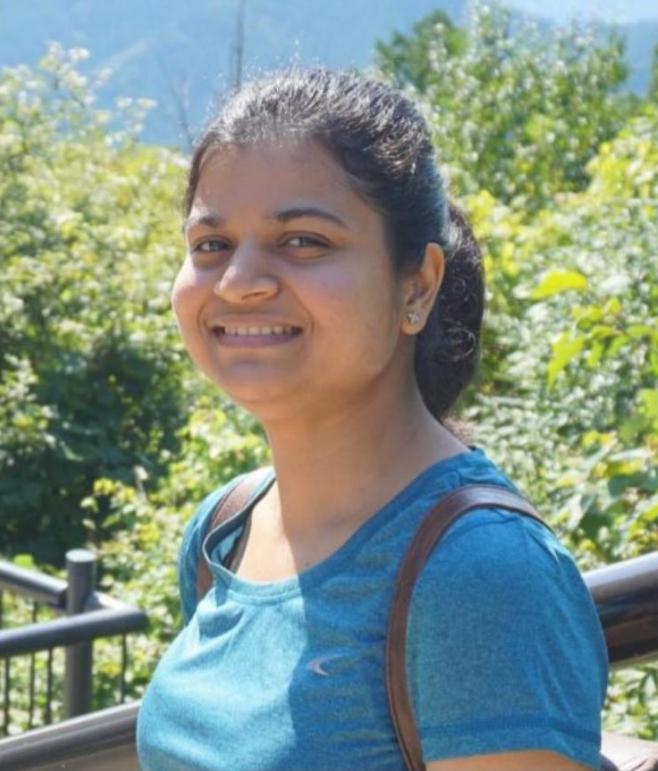
Research Work
My research work is focused on developing neural methods for representation learning of speech and audio signals, with the goal of improving downstream applications that rely on these representations. The key highlights are:
- Identifies two stages of representation learning from raw speech/audio waveform. For each stage, we pursue two broad directions - supervised and unsupervised.
- First part of the thesis work - deals with unsupervised learning of representations. It learns first stage of time-frequency representations from raw waveforms, and second stage of modulation representations that are distinctand irredundant. Uses - RBM, AE, VAE, GAN, skip-connection, residual and modified cost function.
- Second part of the work - deals with supervised 2-stage deep representation learning consisting of a relevanceweighting mechanism. It acts as a feature selection module and weighs the relevance of the acoustic and modulation representations in predicting the target class.
- 2-stage approach may also use target embeddings to learn representation weighting (word2vec style). Theproposed approach is then extended to audio signals for the urban sound classification task. Uses DNN, CNN,LSTM layers with parametric design of layers.
Details
In the first part of the work, we propose representation learning methods for speech data in an unsupervised manner. Using the modulation representation learning as the goal, we explore various neural architecture for unsupervised learning like restricted Boltzmann machines (RBM), variational auto-encoders (VAE) and generative adversarial networks (GAN). For learning modulation representations that are distinct and irredundant, we propose different learning frameworks like external residual approach, skip connection based approach, and cost function based approach. The methods developed for rate and scale representation learning are benchmarked using an automatic speech recognition (ASR) task on noisy and reverberant conditions. We also illustrate that the unsupervised representation learning can be extended to the first stage of learning time-frequency representations from raw waveforms.
The second part of the research work deals with supervised representation learning. Here, we propose a two-stage representation learning approach from raw waveform consisting of acoustic filterbank learning (time-frequency representation learning) from raw waveform followed by a modulation representation learning. The key novelty in the proposed framework consists of a relevance weighting mechanism that acts as a feature selection module. This is inspired by gating networks and provides a mechanism to weight the relevance of the acoustic and modulation representations in predicting the target class. The relevance weighting network can also utilize feedback from the previous predictions of the model for tasks like ASR. The proposed relevance weighting scheme is shown to provide significant performance improvements for ASR task and UrbanSound audio classification task. A detailed analysis yields insights into the interesting properties of the relevance weights that are captured by the model at the acoustic and modulation stages for speech and audio signals.